2024年12月12日(周四) |
8:15-8:40 |
参会嘉宾报到 |
8:40-9:00 |
会议开幕式(主持人:吴鹏 福州大学 教授) 领导致欢迎辞、论坛主席致辞 合影 |
9:00-9:40 |
报告1: 梁哲 (同济大学 教授) 报告题目: 机器学习是复制还是学习,以航班恢复为例 主持人:陈新(佐治亚理工学院 教授) |
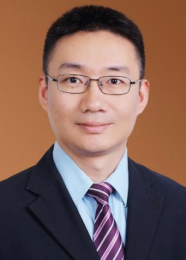
|
摘要:人工智能/机器学习的兴起为求解大规模组合优化问题带来了新的思路。在训练机器学习算法时,一般需要大量实际或模拟数据,机器学习会根据这些训练数据推断新样本的属性。当训练数据中存在与新样本较为一致的场景时,机器学习一般会给出较为准确的判断,我们可以直观地认为机器学习在“复制”训练场景的结果。然而,在实际生产中,很多问题存在数据量不足的特性,且模拟出所有可能发生的场景较难实现或代价高昂。因此,本研究旨在探索机器学习能否根据非全量训练数据,对一些与训练集数据存在显著差异的新场景,给出较好的推断结果。简而言之,机器学习是否真正具有“学习”能力,而不是简单的“复制”。本研究以航班恢复为例,测试了当航空公司网络、航班扰动原因(如机械故障、天气原因)、航班扰动时间、航班扰动机场与训练数据具有较大差异时,机器学习能否根据有限的训练数据给出满意的恢复结果。 |
个人简介:梁哲,国家杰青、首届国家杰青延续项目获得者,同济大学教授。理论研究主要集中在大规模组合优化、整数规划领域;应用研究主要集中在交通、物流特别是航空运营管理领域。在管理科学和交通领域期刊INFORMS Journal on Computing, Transportation Science, European Journal of Operational Research 等发表论文40余篇。团队研发的多个智能航空运营决策系统已经在10余家航司和机场应用,产生了较好的经济效益。 |
9:40-10:20 |
报告2: 龚锡挺(香港中文大学 教授) Talk Title: Managing Perishable Inventory Systems with Positive Lead Times: Inventory Position vs. Projected Inventory Level 主持人:陈新(佐治亚理工学院 教授) |
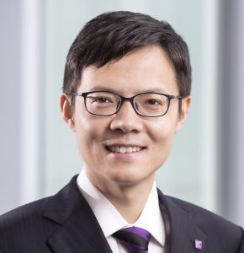
|
Abstract: We consider periodic-review perishable inventory systems with a fixed product lifetime and positive replenishment lead times. Demands are satisfied by on-hand inventories of different ages following a general issuance policy. Unsatisfied demand is either backlogged or lost. The objective is to minimize the long-run average holding, penalty, and outdating costs. The optimal replenishment policy for these systems is notoriously complex and intractable due to the curse of dimensionality. Previous studies have shown that the class of base-stock policies, which maintains a constant inventory position in each period, performs poorly when the unit penalty cost is large. In this paper, we propose a more appealing class of projected inventory level (PIL) policies that maintains a constant expected on-hand inventory level at the time of each order arrival. Among many other results, we prove that for both backlogging and lost-sales systems, the best PIL policy is asymptotically optimal with large unit penalty costs under a large class of demand distributions and with large market sizes under mild conditions. Numerically, the best PIL policy performs close to optimal and much better than the best base-stock policy. This is joint work with Jinzhi Bu (HK PolyU) and Huanyu Yin (CUHK). |
个人简介:龚锡挺现为香港中文大学商学院决策、营运与科技学系教授。在加入香港中文大学之前,他曾在美国密歇根大学工业与运作工程系从事博士后研究。他在北京大学数学科学学院获得学士学位、光华管理学院获得硕士与博士学位。主要研究领域是运营与供应链管理,包括随机库存管理、固定寿命易逝品库存管理、回收再制造生产库存管理、收益管理与定价等。他在Management Science、Operations Research、Production and Operations Management等管理科学领域的国际顶级学术期刊发表了十多篇论文。他主持了多项由香港研究资助委员会资助和一项由华为公司资助的随机库存管理科研项目、并获得国家杰出青年科学基金资助。他目前担任国际学术期刊Naval Research Logistics和Operations Research Letters的副主编。 |
10:20-10:40 |
茶歇 |
10:40-11:20 |
报告3:宋苗(香港理工大学 教授) Talk Title: The Benefits from Service Differentiation: Two-echelon Distribution Network Design with Service Mode Selection 主持人:杨翼(浙江大学 教授) |
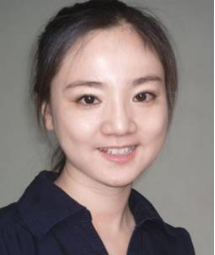
|
Abstract: Classical distribution network design models typically assume exogenous demand and gross profit rate. In practice, however, both demand and profitability depend on various factors such as selling prices, delivery methods, after-sale terms, etc., which are often subject to choices, particularly for companies providing differentiated services. This paper studies the two-echelon distribution network design problem with service differentiation, which is captured by distinct service modes, each could represent any one or any combination of various factors having an impact on demand and profitability. The service modes selection is embedded in network design decisions to simultaneously locate distribution centers (DCs), assign DCs to selected retailers with particular service modes, and replenish inventory for both DCs and retailers, while maximizing the system-wide profit. This problem is formulated as a mixed integer program, which is nonlinear due to the two-echelon inventory replenishment and the impact of service mode selection on demand and profitability. We explore the submodularity of the nonlinear objective function and derive a linearized equivalent reformulation based on the extended polymatroid inequalities. To solve the polymatroid reformulation with exponentially many constraints, we employ a cutting plane approach. The corresponding separation problem is efficiently solved in polynomial time by a tailored algorithm utilizing the structure of its optimal solution. The efficiency of the proposed polymatroid cutting plane algorithm in solving large-size instances is validated in the numerical studies. Through comparisons with benchmarks and sensitivity analysis, the numerical results further demonstrate the benefits from service differentiation in our model and explore the trade-offs between higher and lower degrees of differentiation. |
个人简介:宋苗是香港理工大学物流及航运学系的教授。她分别于西安交通大学、新加坡国立大学及麻省理工学院获得学士、硕士和博士学位。宋苗教授在运筹学方法和运营管理中的应用研究方面取得了一些创新性成果,特别是在物流网络设计和库存优化方面。她曾在Management Science和Operations Research等管理科学领域的国际顶级学术期刊上发表过多篇论文。她的研究项目曾获得国家自然科学基金2019年度优秀青年科学基金项目的资助。 |
11:20-12:00 |
报告4:邓天虎(清华大学 副教授) Talk Title: Conditional Convexity and Its Applications
主持人:杨翼(浙江大学 教授) |
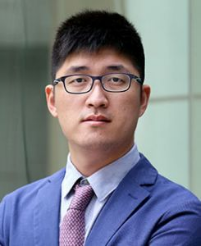
|
Abstract: Inventory models are founded on convexity. When the cost functions are nonconvex, the optimal policy is often deemed untractable. In this paper, we minimize the composition of a convex function and the minimum function, which is generally nonconvex. Firstly, by evenly and uniquely partitioning the two-dimensional Euclidean space into multiple regions, we define a class of functions as conditionally convex if they are convex in each region. Secondly, by requiring coordinate-wise convexity to guarantee that each region’s minimizer is monotone, we characterize the optimal policy as a conditional order-up-to policy, which reduces to the classic order-up-to policy in each region. Thirdly, given the unique partition, we prove the preservation of the proposed properties in minimization problems with fixed and random capacity, which is crucial in multi-period applications. At last, we apply the properties in a two-component assembly system under discrete random capacity. |
个人简介:邓天虎目前是清华大学工业工程系副教授,他2013年于美国加州大学伯克利分校获得工业工程与运筹博士学位,2008年于清华大学工业工程系获得学士学位。他目前研究方向侧重智慧供应链,已经在Operations Research和Manufacturing & Service Operations Management等国际高水平学术期刊和学术会议发表论文20余篇。 |
12:00-13:45 |
午餐 |
14:00-14:40 |
报告5:李梦龙(香港城市大学 助理教授) Talk Title: Online Learning for Dynamic Pricing with Infrequent Inventory Replenishments
主持人:李德彪(福州大学 教授) |
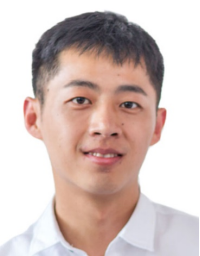
|
Abstract: We consider a joint pricing and inventory control problem where pricing can be adjusted more frequently than inventory ordering decisions. More specifically, the pricing decision is adjusted every period, while new inventories are ordered every epoch that consists of multiple periods. This is motivated by many examples, especially for online retailers, where prices are much easier to change than inventory levels, because changing the latter is subject to prior arrangements or logistic constraints. We consider the situation where the demand-price function and the distribution of random demand noise are both unknown to the retailer, and the retailer needs to develop an online learning algorithm to learn those information and simultaneously maximize total profit. We propose a learning algorithm by applying linear bandit techniques under the upper confidence bound (UCB) framework and prove that the algorithm converges through the DP recursions to approach the optimal pricing and inventory control policy under complete demand information. The theoretical lower bound for the convergence rate of the learning algorithm is proved based on the multivariate Van Trees inequality coupled with some structural DP analyses, and we show that the upper bound of our algorithm’s convergence rate matches the theoretical lower bound. Numerical results show that our learning algorithm performs very well. |
个人简介:李梦龙是香港城市大学商学院管理科学助理教授。在加入香港城市大学之前,他是麻省理工学院数据、系统和社会研究所的博士后助理。他获得了伊利诺伊大学厄巴纳-香槟分校的运筹学博士学位、皮埃尔和玛丽居里大学的数学硕士学位以及清华大学的数学学士学位。他的研究兴趣包括库存管理、收入管理、(离散)凸分析、组合优化、近似算法和数据驱动决策,已经在Operations Research和Production and Operations Management等管理科学领域的国际顶级学术期刊上发表多篇论文。 |
14:40-15:20 |
报告6:王彤(香港城市大学 助理教授) Talk Title: Near-Optimal Dispatch Policies for Emergency Medical Services
主持人:李德彪(福州大学 教授) |
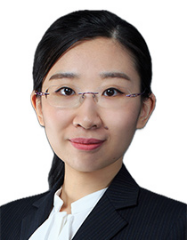
|
Abstract: Emergency medical services (EMS) play a critical role in ensuring timely and effective healthcare delivery, particularly in situations where rapid response is important. The dispatching of ambulances, a fundamental component of EMS, is a complex decision-making process that directly influences patient outcomes. Over the past four decades, the frequency of medical calls has significantly increased. Consequently, cities are faced with the challenge of managing limited resources to respond promptly to current emergency calls while maintaining the capacity to address potential future services. In this paper, we consider the dynamic nature of call arrivals and the inherent heterogeneity in service times across different station-location pairs with general distributions. Our goal is to determine which unit to dispatch to each sequentially observed call to minimize the system-wide average cost. To address this problem, we develop an easy-to-implement policy based on the Lagrangian relaxation for a Markov decision process approximation. Our case study, based on real data from New York City, demonstrates how implementing various proposed policies can effectively reduce costs under different levels of demand. |
个人简介:王彤现为香港城市大学管理科学系助理教授,主要研究方向包括随机建模与优化、强化学习、随机动态规划等,以及他们在供应链与库存管理、动态定价与收益管理、可持续运营管理等方向的应用。研究成果发表在Operations Research,Production and Operations Management等UTD24期刊,主持多项科研基金项目,包括国家自然科学基金优秀青年项目、上海市浦江人才计划项目等。 |
15:20-15:40 |
茶歇 |
15:40-16:20 |
报告7:高品(香港中文大学(深圳) 助理教授) Talk Title: Assortment Optimization with Downward Feasibility: Efficient Heuristics Based on Independent Demands
主持人:谢维(福州大学 教授) |
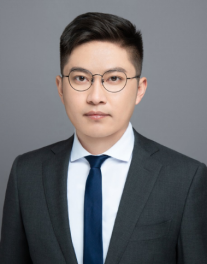
|
Abstract: One of the key challenges in revenue management is to find a subset of products or services to offer customers to maximize the expected revenue of a firm. Often, the set of feasible assortments must satisfy multiple business constraints. In this paper, we develop a general heuristic for the assortment optimization problem that can be applied to various choice models. Our investigation reveals that while optimizing assortment decisions needs to account for substitution effects among products, we advocate simplifying the problem to one akin to the independent demand model, where the demand for a product is independent of other offered products, and all product revenues are uniformly reduced by a constant. The heuristic requires minimal information about the underlying choice model, specifically only the last-choice probabilities, which are defined as the purchase probabilities when the assortment consists of only one product. For last-choice regular models that satisfy certain bounding conditions, our heuristic achieves the best possible performance guarantees and necessitates solving only two linear programs when the constraints are totally unimodular (TUM) and downward feasible. More generally, we propose heuristic variants for cases where constraints are not TUM or the bounding conditions are difficult to determine. Leveraging the proposed methodology, we develop nontrivial heuristics for several previously studied problems, either by relaxing the assumption or improving the approximation ratio. Multiple computational experiments show that our heuristics exhibit exceptional performance. Furthermore, an analysis of a real-world dataset demonstrates that our heuristics, when derived from estimated models with higher predictive accuracy, achieve superior optimization outcomes. |
个人简介:高品为香港中文大学(深圳)助理教授,他于2021年获得香港科技大学的博士学位。他的研究兴趣包括新兴商业模式中的收益管理和运营管理,已在Operations Research、Management Science和Manufacturing & Service Operations Management等国际顶级学术期刊上发表过多篇论文。他曾获得多个权威研究奖项,包括 ISCOM 最佳论文奖(第一名)和 POMS-China 最佳论文奖(第二名)。 |
16:20-17:00 |
报告8:姜嘉硕(香港科技大学 助理教授) Talk Title: Online Stochastic Optimization with Wasserstein Based Non-stationarity
主持人:谢维(福州大学 教授) |
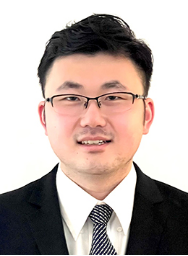
|
Abstract: We consider a general online stochastic optimization problem with multiple budget constraints over a horizon of finite time periods. In each time period, a reward function and multiple cost functions are revealed, and the decision maker needs to specify an action from a convex and compact action set to collect the reward and consume the budget. Each cost function corresponds to the consumption of one budget. In each period, the reward and cost functions are drawn from an unknown distribution, which is non-stationary across time. The objective of the decision maker is to maximize the cumulative reward subject to the budget constraints. This formulation captures a wide range of applications including online linear programming and network revenue management, among others. In this paper, we consider two settings: (i) a data-driven setting where the true distribution is unknown but a prior estimate (possibly inaccurate) is available; (ii) an uninformative setting where the true distribution is completely unknown. We propose a unified Wasserstein-distance based measure to quantify the inaccuracy of the prior estimate in setting (i) and the non-stationarity of the system in setting (ii). We show that the proposed measure leads to a necessary and sufficient condition for the attainability of a sublinear regret in both settings. For setting (i), we propose a new algorithm, which takes a primal-dual perspective and integrates the prior information of the underlying distributions into an online gradient descent procedure in the dual space. The algorithm also naturally extends to the uninformative setting (ii). Under both settings, we show the corresponding algorithm achieves a regret of optimal order. In numerical experiments, we demonstrate how the proposed algorithms can be naturally integrated with the re-solving technique to further boost the empirical performance. |
个人简介:姜嘉硕现为香港科技大学工业工程与决策分析系助理教授。他于2022 年获得纽约大学斯特恩商学院运营管理博士学位。主要研究领域是运营管理、运筹学、收入和社会福利优化、随机优化、供应链管理等。他在Management Science、Operations Research等管理科学领域的国际顶级学术期刊发表多篇论文。他主持和参与了多项由香港研究资助委员会资助的资源分配管理科研项目。 |
17:30-19:30 |
晚餐 |